

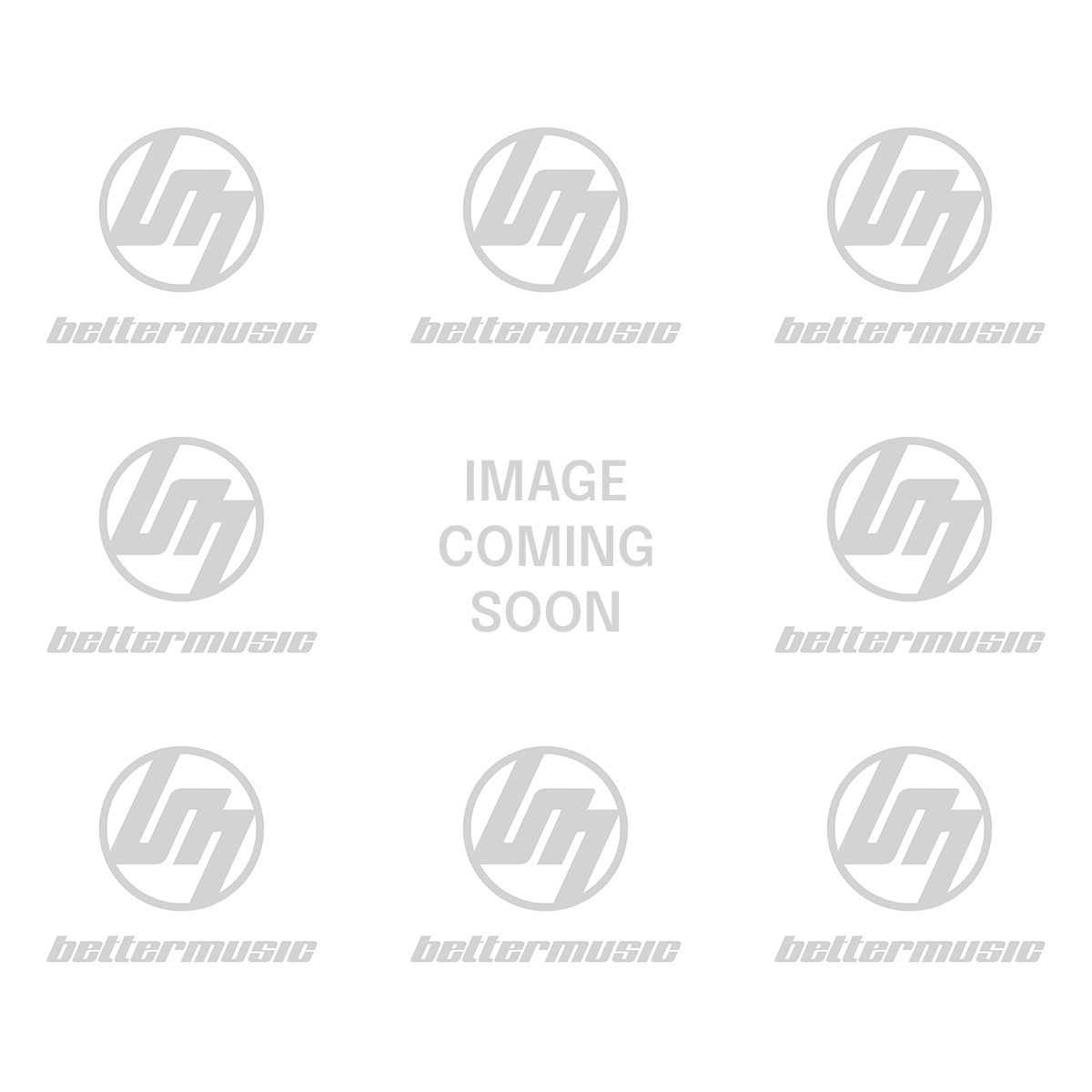
One such promising algorithm is Alternating Direction Method of Multipliers (ADMM). While progress has been made in adapting existing algorithms to these difficult settings, the issues of computational cost and convergence guarantees still remain. The issue is that cost functions for physics and machine learning applications are challenging for many standard minimization algorithms, which make often convexity and smoothness assumptions about the problem. In typical inverse problems, one minimizes some cost/data misfit function to find model parameters that effectively capture the collected data.
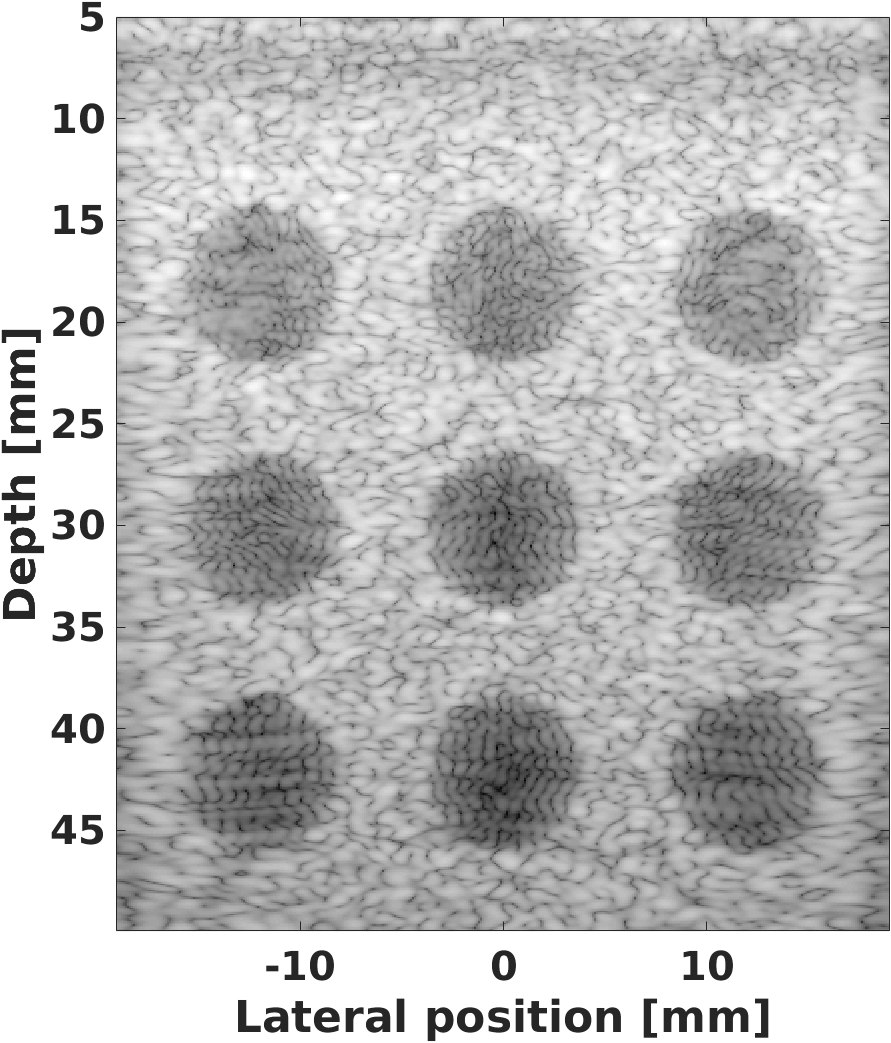
This practicum will focus on developing a novel method for problems with these characteristics and then explore the descent and convergence properties of this algorithm in the context of the aforementioned settings. Examples of these are the nonsmooth regularization of generalized lasso, low-rank regularized matrix completion, sparse convolutional neural networks, and physics-based, PDE-constrained optimization. Large, nonconvex, and nonlinear problems arise naturally in physical inverse problems and machine learning applications.
